英文原文
There are n
cities numbered from 0
to n-1
. Given the array edges
where edges[i] = [fromi, toi, weighti]
represents a bidirectional and weighted edge between cities fromi
and toi
, and given the integer distanceThreshold
.
Return the city with the smallest number of cities that are reachable through some path and whose distance is at most distanceThreshold
, If there are multiple such cities, return the city with the greatest number.
Notice that the distance of a path connecting cities i and j is equal to the sum of the edges' weights along that path.
Example 1:
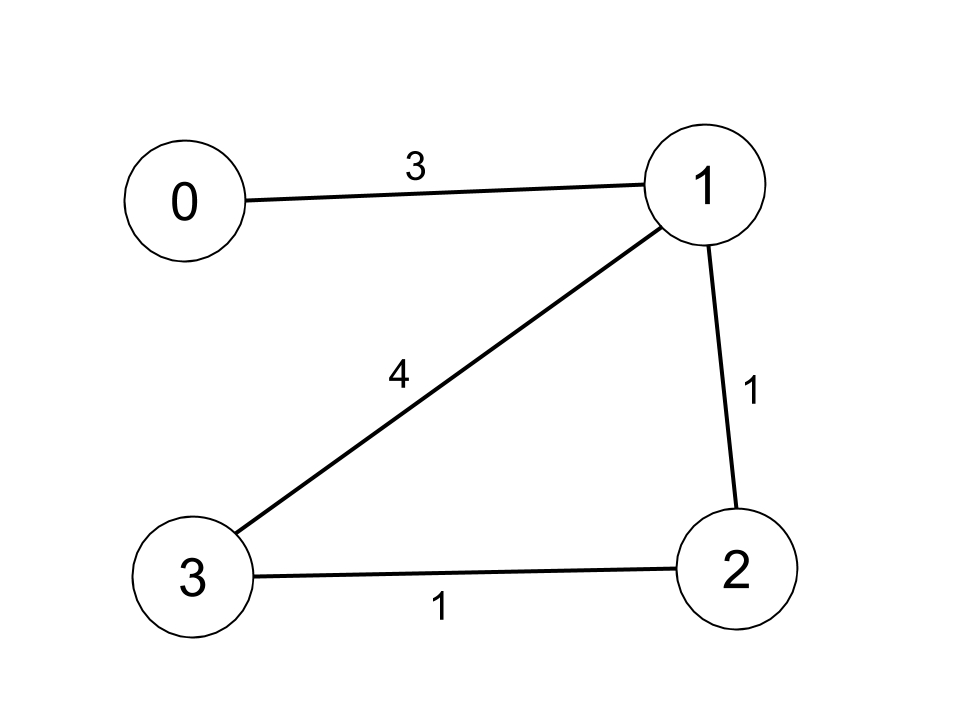
Input: n = 4, edges = [[0,1,3],[1,2,1],[1,3,4],[2,3,1]], distanceThreshold = 4 Output: 3 Explanation: The figure above describes the graph. The neighboring cities at a distanceThreshold = 4 for each city are: City 0 -> [City 1, City 2] City 1 -> [City 0, City 2, City 3] City 2 -> [City 0, City 1, City 3] City 3 -> [City 1, City 2] Cities 0 and 3 have 2 neighboring cities at a distanceThreshold = 4, but we have to return city 3 since it has the greatest number.
Example 2:
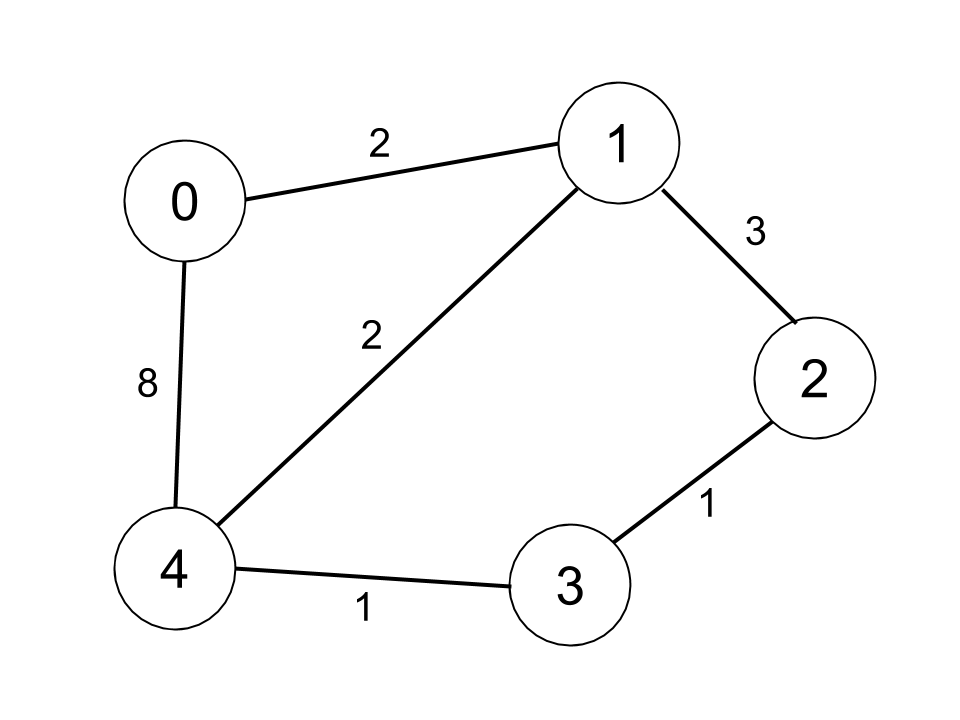
Input: n = 5, edges = [[0,1,2],[0,4,8],[1,2,3],[1,4,2],[2,3,1],[3,4,1]], distanceThreshold = 2 Output: 0 Explanation: The figure above describes the graph. The neighboring cities at a distanceThreshold = 2 for each city are: City 0 -> [City 1] City 1 -> [City 0, City 4] City 2 -> [City 3, City 4] City 3 -> [City 2, City 4] City 4 -> [City 1, City 2, City 3] The city 0 has 1 neighboring city at a distanceThreshold = 2.
Constraints:
2 <= n <= 100
1 <= edges.length <= n * (n - 1) / 2
edges[i].length == 3
0 <= fromi < toi < n
1 <= weighti, distanceThreshold <= 10^4
- All pairs
(fromi, toi)
are distinct.
中文题目
有 n
个城市,按从 0
到 n-1
编号。给你一个边数组 edges
,其中 edges[i] = [fromi, toi, weighti]
代表 fromi
和 toi
两个城市之间的双向加权边,距离阈值是一个整数 distanceThreshold
。
返回能通过某些路径到达其他城市数目最少、且路径距离 最大 为 distanceThreshold
的城市。如果有多个这样的城市,则返回编号最大的城市。
注意,连接城市 i 和 j 的路径的距离等于沿该路径的所有边的权重之和。
示例 1:
输入:n = 4, edges = [[0,1,3],[1,2,1],[1,3,4],[2,3,1]], distanceThreshold = 4 输出:3 解释:城市分布图如上。 每个城市阈值距离 distanceThreshold = 4 内的邻居城市分别是: 城市 0 -> [城市 1, 城市 2] 城市 1 -> [城市 0, 城市 2, 城市 3] 城市 2 -> [城市 0, 城市 1, 城市 3] 城市 3 -> [城市 1, 城市 2] 城市 0 和 3 在阈值距离 4 以内都有 2 个邻居城市,但是我们必须返回城市 3,因为它的编号最大。
示例 2:
输入:n = 5, edges = [[0,1,2],[0,4,8],[1,2,3],[1,4,2],[2,3,1],[3,4,1]], distanceThreshold = 2 输出:0 解释:城市分布图如上。 每个城市阈值距离 distanceThreshold = 2 内的邻居城市分别是: 城市 0 -> [城市 1] 城市 1 -> [城市 0, 城市 4] 城市 2 -> [城市 3, 城市 4] 城市 3 -> [城市 2, 城市 4] 城市 4 -> [城市 1, 城市 2, 城市 3] 城市 0 在阈值距离 2 以内只有 1 个邻居城市。
提示:
2 <= n <= 100
1 <= edges.length <= n * (n - 1) / 2
edges[i].length == 3
0 <= fromi < toi < n
1 <= weighti, distanceThreshold <= 10^4
- 所有
(fromi, toi)
都是不同的。
通过代码
高赞题解
$Floyd$ 算法
$Floyd$ 算法又称插点法,其中算法的核心思想是动态规划。
算法步骤
通过已知条件初始化距离矩阵 $D[n][n]$ ,其中 $D[i][j]$ 表示,顶点 $i$ 到顶点 $j$ 的距离。
$n$ 个顶点依次作为插入点,例如,$k$ 为其中一个顶点,$D[i][k] + D[k][j] < D[i][j]$ ,那说明顶点 $i$ 经过顶点 $k$ 再到达 $j$ ,比直接到达 $j$ 要近。所以更新 $D[i][j]:$ $D[i][j] = D[i][k] + D[k][j]$ 。
可以归纳得到状态转移方程:$D[i][j] = min(D[i,k]+D[k,j],D[i,j])$ 。
$Floyd$ 核心代码:
// Floyd算法
for (int k = 0; k < n; k++) {
// n个顶点依次作为插入点
// 注意插点k是放在第一层循环,后面会解释原因
for (int i = 0; i < n; i++) {
for (int j = 0; j < n; j++) {
// 遍历各个顶点之间的距离,并用插入点进行更新
D[i][j] = min(D[i][k]+D[k][j], D[i][j]);
}
}
}
题目解析
使用 $Floyd$ 算法求出各个城市到其它城市的距离,保存在矩阵 $D[n][n]$ 中。
遍历 $D[n][n]$ ,统计各个城市在距离不超过 $distanceThreshold$ 的情况下,能到达的其它城市的数量。
返回能到达其它城市最少的城市 $ret$ 。
解题代码
class Solution {
public:
int findTheCity(int n, vector <vector<int>> &edges, int distanceThreshold) {
// 定义邻接矩阵D,并初始化各个城市间距离为INT_MAX(无穷)
vector <vector<int>> D(n, vector<int>(n, INT_MAX));
// 根据edges[][]初始化D[][]
for (auto &e : edges) {
// 无向图两个城市间的两个方向距离相同
D[e[0]][e[1]] = e[2];
D[e[1]][e[0]] = e[2];
}
// Floyd算法
for (int k = 0; k < n; k++) {
// n个顶点依次作为插入点
for (int i = 0; i < n; i++) {
for (int j = 0; j < n; j++) {
if (i == j || D[i][k] == INT_MAX || D[k][j] == INT_MAX) {
// 这些情况都不符合下一行的if条件,
// 单独拿出来只是为了防止两个INT_MAX相加导致溢出
continue;
}
D[i][j] = min(D[i][k] + D[k][j], D[i][j]);
}
}
}
// 选择出能到达其它城市最少的城市ret
int ret;
int minNum = INT_MAX;
for (int i = 0; i < n; i++) {
int cnt = 0;
for (int j = 0; j < n; j++) {
if (i != j && D[i][j] <= distanceThreshold) {
cnt++;
}
}
if (cnt <= minNum) {
minNum = cnt;
ret = i;
}
}
return ret;
}
};
答疑:为什么遍历插入点k是放在第一层循环?
这个源自 $Floyd$ 的核心思想–动态规划,代码中的二维状态转移方程 $D[i][j] = min(D[i,k]+D[k,j],D[i,j]);$ ,其实是从三维简化得到的。
我们不妨从最初的三维说起:
首先定义状态数组(也就是距离矩阵)$D[n][n][n]$,其中 $D[k][i][j]$ 表示顶点 $i$, 顶点 $j$ 通过前 $k$ 个顶点得到的最短距离。
$D[k][i][j]$ 是从 $D[k-1][i][j]$ 和 $D[k-1][i][k] + D[k-1][k][j]$ 两者中值较小的一个转移得到的,也就是说要在前 $k-1$ 个顶点已经插入,更新距离矩阵状态之后,第 $k$ 个顶点才能作为插入顶点。
归纳得到状态转移方程:$D[k][i][j] = min(D[k-1][i][j], D[k-1][i][k] + D[k-1][k][j])$。
其中 $k$ 的作用是标志到达了第几个插入点,也就是状态数组到达了哪个状态,不用刻意记录,于是减去第一维就变成了二维。
明白了 $Floyd$ 的三维 $dp$ 思想,根据状态转移方程在编码时就很自然的会将 $k$ 放在第一层循环,而将 $k$ 放在最后一层则是错误的编码。
补充一个 $dijkstra$ 的代码
class Solution {
public:
void dijkstra(int graph[101][101], int dist[101], int v, int n) {
// 初始化 dist
for (int i = 0; i < n; i++) {
dist[i] = graph[v][i];
}
// 标记当前点是否已经加入集合
bool m[101];
memset(m, 0, sizeof(m));
m[v] = true;
// 每次都找离集合最近的一个点,一共要找 n-1 次
for (int k = 0; k < n-1; k ++) {
int minv = INT_MAX;
int next = v;
for (int i = 0; i < n; i ++) {
if (!m[i] && dist[i] < minv) {
minv = dist[i];
next = i;
}
}
m[next] = true;
// 每次找到最近的点,并用它更新其它点到集合的距离,
// 也就是更新dist数组
for (int i = 0; i < n; i ++) {
if (m[i] || graph[next][i] == INT_MAX) {
continue;
}
dist[i] = min(dist[i], dist[next] + graph[next][i]);
}
}
}
int findTheCity(int n, vector<vector<int>>& edges, int distanceThreshold) {
// 定义并初始化邻接矩阵 graph
int graph[101][101];
for (int i = 0; i < n; i ++) {
for (int j = 0; j < n; j ++) {
graph[i][j] = INT_MAX;
}
}
for (auto& edge : edges) {
graph[edge[0]][edge[1]] = edge[2];
graph[edge[1]][edge[0]] = edge[2];
}
int mincnt = INT_MAX;
int ret = 0;
for (int i = 0; i < n; i ++) {
// 使用单源最短路径dijkstra算法生成:
// 以i为源点到其它各点的最短路径数组dist
int dist[101];
dijkstra(graph, dist, i, n);
int cnt = 0;
for (int j = 0; j < n; j ++) {
if (dist[j] <= distanceThreshold) {
cnt ++;
}
}
if (cnt <= mincnt) {
mincnt = cnt;
ret = i;
}
}
return ret;
}
};
最后
感谢您的观看!欢迎大家留言,一起讨论交流。
统计信息
通过次数 | 提交次数 | AC比率 |
---|---|---|
6073 | 12226 | 49.7% |
提交历史
提交时间 | 提交结果 | 执行时间 | 内存消耗 | 语言 |
---|